Define Generative AI and Differentiate between Generative & Conventional AI
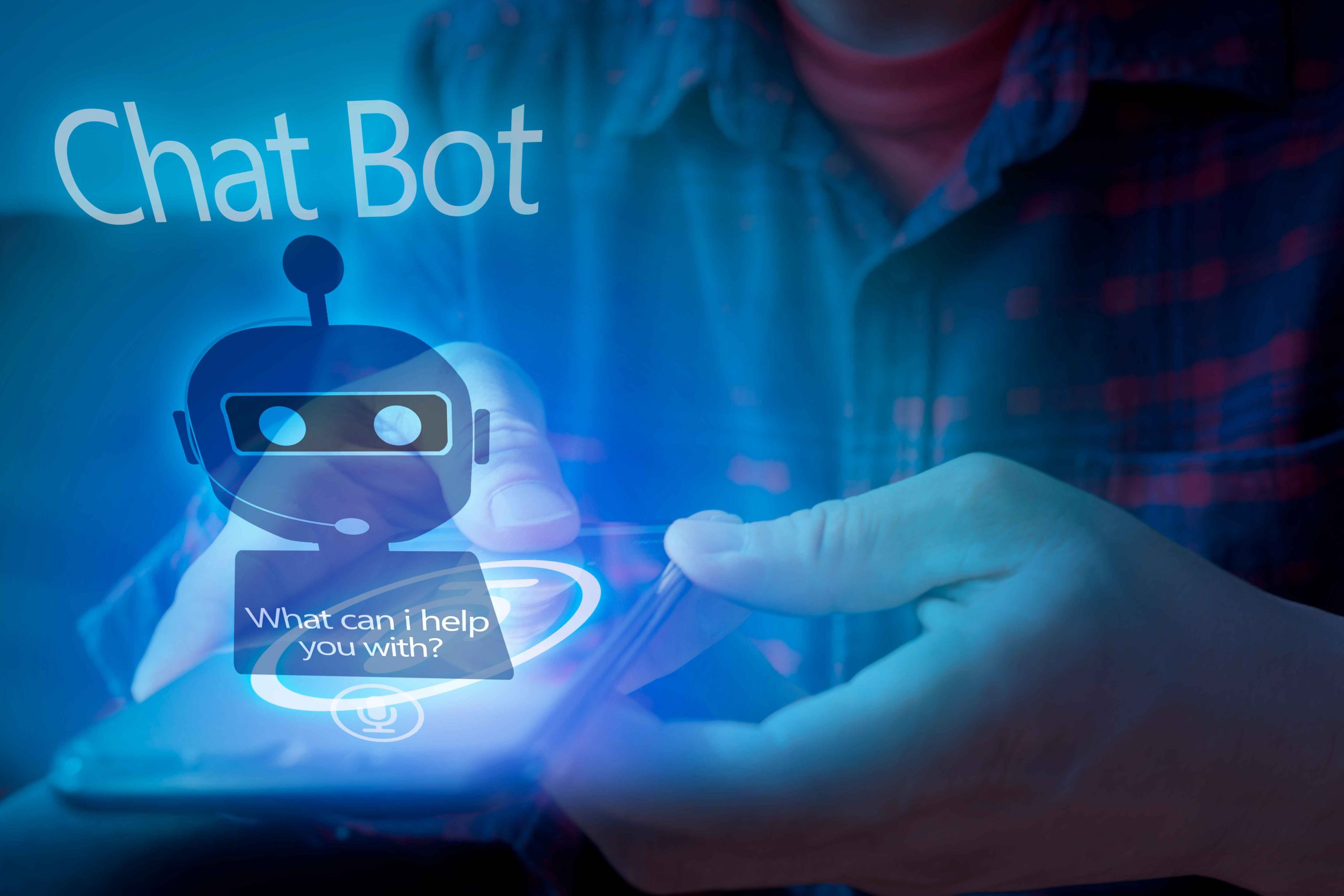
Generative AI:
Generative AI refers to a class of artificial intelligence models and algorithms designed to generate new, original data that resembles and is similar to the data it was trained on. These models can create new content by learning patterns and structures from existing datasets, such as images, text, audio, and more. Generative AI technologies, like Generative Adversarial Networks (GANs), Variational Autoencoders (VAEs), and Recurrent Neural Networks (RNNs), are widely used in creative applications, content generation, data augmentation, and synthetic data creation.
Differentiate between Generative AI & Conventional AI
The primary distinction between Generative AI and Conventional AI lies in their main objectives and capabilities:
Objective:
Generative AI: The main goal of Generative AI is to produce new, original data that is similar to the training data, enabling the creation of synthetic content and generating novel outputs.
Conventional AI: Conventional AI aims to solve specific problems and make decisions based on existing data. It focuses on learning patterns from data to perform specific tasks, such as classification, regression, or decision-making.
Applications:
Generative AI: Generative AI finds applications in creative fields, content generation, data augmentation, style transfer, and other areas where creating new content is essential.
Conventional AI: Conventional AI is widely used in various industries for tasks like image recognition, natural language processing, sentiment analysis, and fraud detection, focusing on making accurate predictions and decisions based on given data.
Output:
Generative AI: The output of Generative AI is new and original data that did not exist in the training dataset, allowing for the generation of realistic and diverse content.
Conventional AI: The output of Conventional AI is typically a specific prediction, classification, or decision based on the input data, with a focus on accuracy and performance for predefined tasks.
Real World Applications of Generative AI
Generative AI has a wide range of real-world applications that span various industries and domains. Some of the prominent real-world applications of Generative AI include:
- Content Generation: Generative AI creates realistic and diverse content, including images, artwork, music, and videos. It finds applications in creative industries, advertising, and content production.
- Data Augmentation: Generative AI is employed to generate synthetic data that can be used to augment existing datasets for training machine learning models, improving model performance and generalization.
- Style Transfer: Generative AI can transfer the style of one image or artwork onto another, enabling artists and designers to experiment with different visual styles easily.
- Text Generation: Generative AI models can generate human-like text, making it useful for chatbots, virtual assistants, and content generation in natural language processing applications.
- Image-to-Image Translation: Generative AI can convert images from one domain to another, such as turning satellite images into maps or black-and-white images into color.
- Medical Image Analysis: Generative AI enhances medical imaging tasks, like denoising, super-resolution, and synthesizing realistic medical images, assisting in diagnosis and treatment planning.
- Drug Discovery: Generative AI is crucial in designing and generating molecular structures for potential drugs, accelerating the drug discovery.
- Virtual Avatars and Characters: Generative AI creates lifelike virtual avatars and characters for video games, virtual reality, and animated movies.
- Financial Market Forecasting: Generative AI models can generate synthetic financial market data to aid in forecasting and simulating market scenarios.
- Architecture and Design: Generative AI can assist architects and designers in creating innovative building designs and structures.
- Fashion and Design: Generative AI is employed in fashion design to create new clothing designs and patterns.
- Video Game Design: Generative AI is used in development for generating game levels, characters, and landscapes.
- Anomaly Detection: Generative AI can be applied in anomaly detection to identify unusual patterns or behaviors in data.
- Speech Synthesis: Generative AI models can generate human-like speech, contributing to text-to-speech and voice assistant applications.
- Robotics and Simulation: Generative AI can be used to generate synthetic training data for robot simulations and reinforcement learning tasks.
These real-world applications demonstrate the versatility and potential impact of Generative AI across various industries, transforming how content is created, data is analyzed, and innovative solutions are developed.
How does Generative AI function?
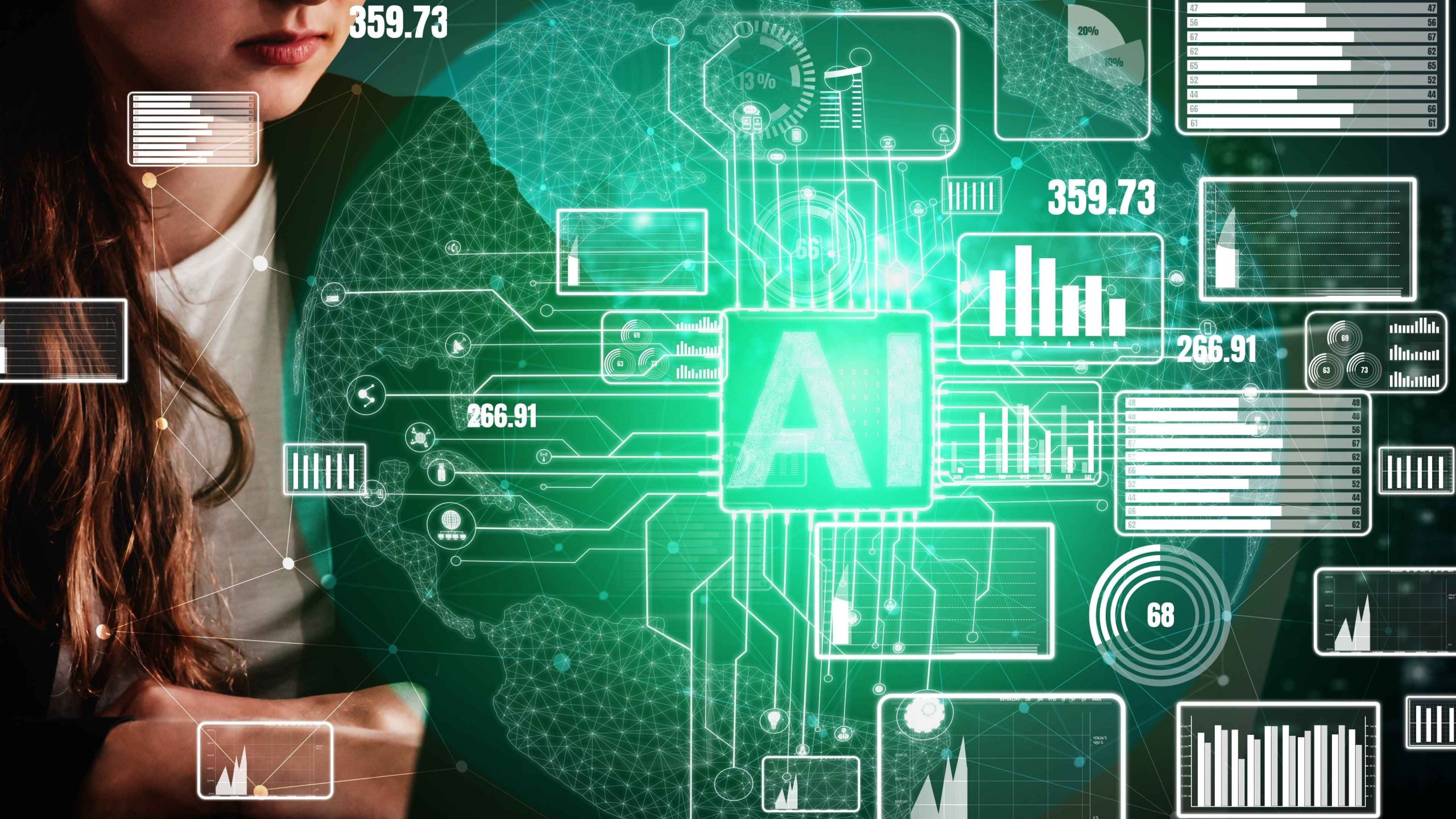
Generative AI functions using advanced machine learning algorithms and models to generate new data closely resembling the patterns and characteristics of the training data it was exposed to. The key components and steps involved in the functioning of Generative AI are as follows:
- Data Collection: The process begins with collecting a large and diverse dataset relevant to the specific domain or task. This dataset serves as the training data for the Generative AI model.
- Training the Model: Generative AI models, such as Generative Adversarial Networks (GANs) or Variational Autoencoders (VAEs), are then trained on the collected dataset. During training, the model learns to capture the underlying patterns and structures present in the data.
- Generating New Data: Once the Generative AI model is trained, it can generate new data instances that resemble the patterns observed in the training data. For example, in the case of GANs, the model consists of two components: a generator and a discriminator. The generator generates new data samples, while the discriminator evaluates whether the generated data is real (from the training data) or fake (caused by the generator). The generator continuously improves its ability to produce realistic data by learning from the feedback provided by the discriminator.
- Feedback Loop and Iteration: The process of generating new data and improving the model’s performance continues through a feedback loop. The model iteratively refines its generation process based on the feedback from its evaluation component (e.g., the discriminator in GANs).
- Diversity and Creativity: Generative AI models are designed to produce diverse and creative outputs. They can generate novel images, text, music, and more that were not present in the original training data but still resemble the characteristics of the data.
- Application-Specific Adaptation: Generative AI models can be fine-tuned and adapted for specific applications and tasks. For instance, they can be trained on specific datasets or incorporate constraints to generate data with particular attributes or styles.
Validation and Testing: After the generative model is trained, it undergoes validation and testing to ensure that the generated data meets the desired quality and usefulness for the intended application.
Benefits of Generative AI
Generative AI offers numerous benefits across various industries and applications. Some of the key advantages of Generative AI include:
- Content Generation: Generative AI can automatically create realistic and diverse content, such as images, artwork, music, and text, saving time and resources for creative tasks.
- Data Augmentation: By generating synthetic data, Generative AI helps in augmenting existing datasets, improving the performance and generalization of machine learning models.
- Personalization: Generative AI enables personalized user experiences by creating content tailored to individual preferences, enhancing customer engagement and satisfaction.
- Creative Exploration: Artists, designers, and developers can use Generative AI to explore new creative ideas and styles, leading to innovative designs and concepts.
- Data Privacy: Generative AI can generate synthetic data, ensuring privacy protection and compliance while maintaining the utility of the data for research and analysis.
- Drug Discovery: In the pharmaceutical industry, Generative AI aids in designing new molecular structures for potential drugs, accelerating drug discovery processes.
- Image-to-Image Translation: Generative AI can translate images from one domain to another, useful in various applications, such as image enhancement and style transfer.
- Realistic Simulation: Generative AI can simulate real-world scenarios, useful in training autonomous vehicles, robotics, and other complex systems.
- Enhanced Decision-Making: Generative AI generates large and diverse datasets and assists in data-driven decision-making and scenario analysis.
- Reduced Data Collection Efforts: Generative AI reduces the need for massive amounts of data collection by generating synthetic data that is similar to the real data.
- Cost Savings: Generative AI can lead to cost savings in content creation, data collection, and data labeling processes.
- Increased Efficiency: Automating content and data generation tasks with Generative AI improves operational efficiency and reduces manual efforts.
- Adversarial Defense: Generative AI models can be used to defend against adversarial attacks by generating robust data samples for training classifiers.
- Data Imputation: Generative AI can fill in missing data points, imputing values in incomplete datasets and enhancing data completeness and accuracy.
- Creative Advertisement and Marketing: Generative AI facilitates the creation of personalized and creative ads and marketing content, improving campaign effectiveness.
Generative AI’s ability to generate realistic and diverse data, content, and experiences offers transformative solutions to various industries, empowering businesses to be more creative, efficient, and innovative.
Technologies Used in Generative AI
Generative AI leverages various cutting-edge technologies to achieve its capabilities. Some of the key technologies used in Generative AI include:
- Generative Adversarial Networks (GANs): GANs consist of two neural networks, a generator and a discriminator, engaged in a competitive process. The generator creates new data samples, while the discriminator differentiates between real and generated data. This competition leads to the generation of highly realistic and diverse outputs.
- Variational Autoencoders (VAEs): VAEs are generative models that encode input data into a low-dimensional latent space and then decode it back to reconstruct the original data. This process allows for continuous and structured data representation, enabling smooth interpolation between data points.
- Recurrent Neural Networks (RNNs): RNNs generate data sequences like text or music. They have a feedback loop that allows them to maintain the memory of past inputs, making them suitable for tasks that require context and sequential dependencies.
- Transformers: Transformers are attention-based neural networks that have revolutionized natural language processing tasks. They capture long-range dependencies and have been widely adopted for text generation and translation.
- Autoencoders: Autoencoders are used for unsupervised learning and feature extraction. They encode input data into a compressed representation and then decode it back to reconstruct the original data. Variations of autoencoders, such as denoising autoencoders, can be used in Generative AI for data reconstruction and generation.
- Deep Reinforcement Learning (RL): Deep RL algorithms, such as Proximal Policy Optimization (PPO) and Deep Q-Networks (DQNs), can be employed in Generative AI for tasks that involve sequential decision-making, learning from interactions with the environment.
- Convolutional Neural Networks (CNNs): CNNs are commonly used in Generative AI for tasks involving image generation and image-to-image translation. They excel at capturing spatial patterns and hierarchical features in visual data.
- Attention Mechanisms: Attention mechanisms, like those used in transformers, enable the model to focus on specific parts of the input data, making them effective for generating contextual and coherent outputs.
- Probabilistic Graphical Models: Probabilistic graphical models, such as Bayesian networks and Markov Random Fields (MRFs), are used to model complex probabilistic relationships in data and are useful in probabilistic generative modeling.
- Monte Carlo Methods: Monte Carlo methods, like Markov Chain Monte Carlo (MCMC), are used in sampling-based generative models to estimate data distributions and perform sampling for generating new data.
Integration of Generative AI into Existing Systems and Workflows
Integrating generative AI into existing systems and workflows requires careful planning and consideration. The following steps outline how generative AI can be effectively integrated:
- Identify Use Cases: Identify specific use cases within your organization where generative AI can add value. Determine areas where content generation, data augmentation, or creative tasks can be enhanced through generative models.
- Data Preparation: Ensure that the data used to train the generative AI models is high quality, diverse, and representative of the target domain. Clean and preprocess the data to remove noise and inconsistencies.
- Model Selection: Choose the appropriate generative AI model based on the identified use case and data characteristics. Consider using pre-trained models as a starting point to speed up development.
- Customization: Fine-tune the generative AI model to suit your organization’s needs and requirements. Customization may involve adjusting hyperparameters, modifying the architecture, or training on domain-specific data.
- Scalability and Performance: Consider the scalability and performance requirements of the generative AI system. Optimize the model to run efficiently on the existing infrastructure and to handle the expected workload.
- API Integration: Many generative AI platforms offer APIs that can be integrated into existing systems and workflows. These APIs allow easy access to the generative AI capabilities from other applications and services.
- User Interface (UI) Integration: If the generative AI system involves user interaction, design a user-friendly interface that seamlessly integrates with existing applications. This ensures smooth user experiences and easy adoption.
- Security and Compliance: Pay close attention to data security and compliance, especially if the generative AI system involves sensitive information. Implement measures to safeguard data and ensure compliance with relevant regulations.
- Testing and Validation: Thoroughly test and validate the integrated generative AI system to ensure its accuracy, reliability, and performance in real-world scenarios.
- User Training and Support: Provide adequate training and support to users who will be interacting with the generative AI system. Familiarize them with its functionalities and guide them on best practices.
- Feedback Loop: Establish a feedback loop to improve the generative AI system continuously. Gather user feedback and monitor performance metrics to identify areas for enhancement and refinement.
- Documentation and Knowledge Sharing: Document the integration process and share knowledge within the organization to facilitate future updates, maintenance, and improvements.
By following these steps, generative AI can seamlessly integrate into existing systems and workflows, empowering organizations to leverage the transformative capabilities of this technology efficiently and effectively.
Limitations and Challenges in Generative AI Research and Development
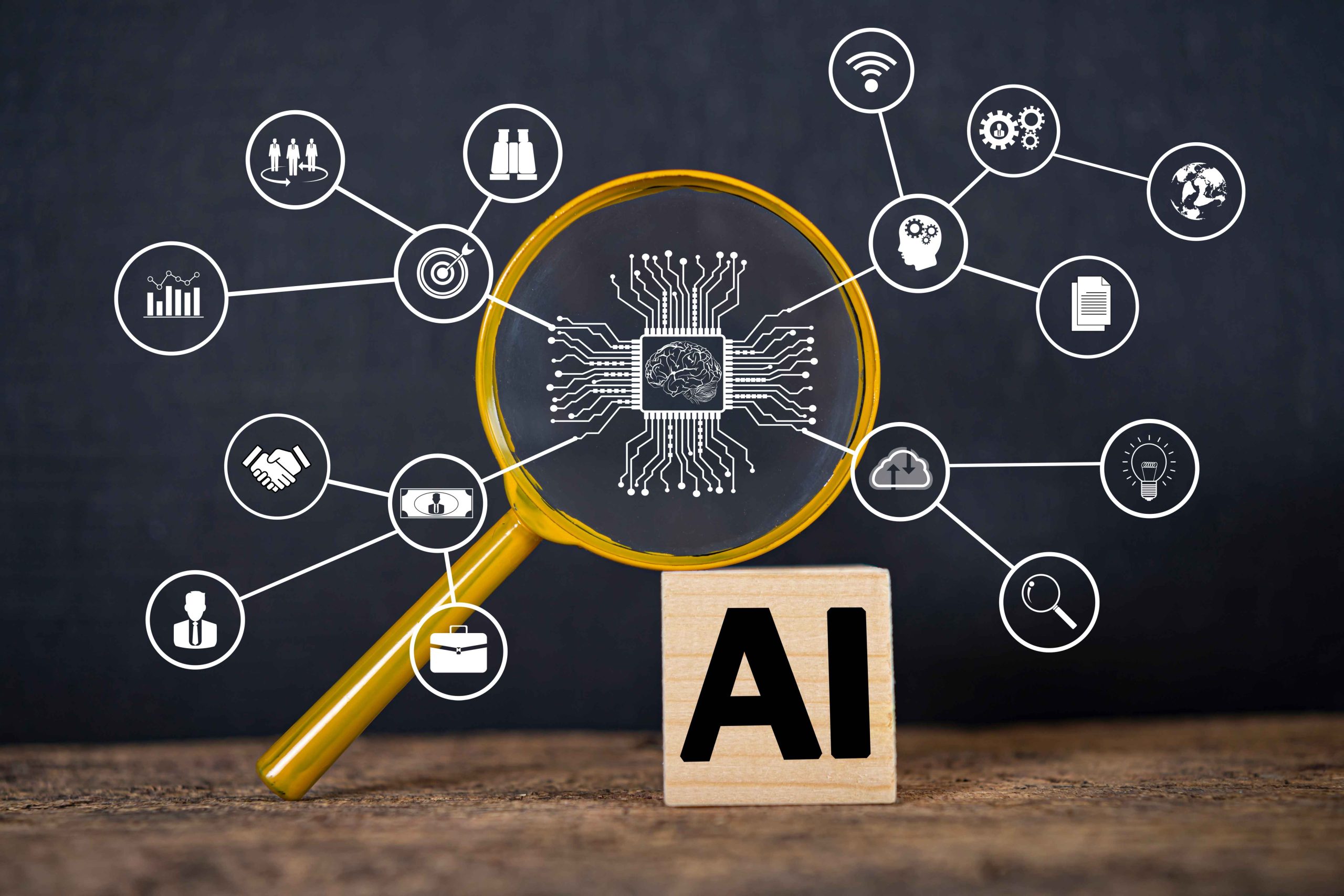
Generative AI research and development have made remarkable progress, but there are several current limitations and challenges that researchers and developers continue to face:
- Quality and Realism: Ensuring the generated content’s quality and realism remains challenging. While generative AI models can produce impressive outputs, achieving consistently high-quality and indistinguishable results from real data is still a work in progress.
- Training Data Requirements: Generative AI models often require large and diverse datasets for training, making data collection and labeling efforts resource-intensive and challenging, particularly in specialized domains with limited data availability.
- Mode Collapse: Some generative AI models, particularly GANs, can suffer from mode collapse, where they produce limited variations of the data and fail to capture the full diversity of the target distribution.
- Generalization: Ensuring that generative AI models generalize well to unseen data and diverse scenarios is challenging. Models may need help generating novel data instances outside the training data distribution.
- Computational Resources: Many advanced generative AI models are computationally demanding, requiring high-performance hardware and significant computational resources for training and inference.
- Interpretability: Understanding how generative AI models arrive at their outputs is challenging, as they often need more interpretability, making it difficult to explain the generation process.
- Ethical Concerns: Generative AI’s ability to produce realistic content raises ethical concerns, such as the creation of deepfakes and misinformation. Addressing the potential misuse and establishing ethical guidelines is critical.
- Training Stability: Training generative AI models can be sensitive to hyperparameters, architecture choices, and the quality of training data, leading to instability and challenging convergence.
- Bias and Fairness: Generative AI models can inadvertently inherit biases in the training data, leading to biased outputs. Ensuring fairness and mitigating bias in generative models is an ongoing area of research.
- Dataset Privacy: Generating synthetic data for privacy protection can be challenging since generative AI models may inadvertently memorize or reveal sensitive information from the training data.
- Domain Adaptation: Adapting generative AI models to new domains or tasks without extensive retraining remains challenging, especially when target domains have limited data.
- Real-Time Generation: Achieving real-time or interactive generation of complex content, such as high-resolution images or 3D models, remains computationally demanding and may require further optimization.
Despite these challenges, ongoing research efforts continuously address these limitations, pushing the boundaries of generative AI technology and opening up exciting possibilities for its real-world applications.